Table Of Content
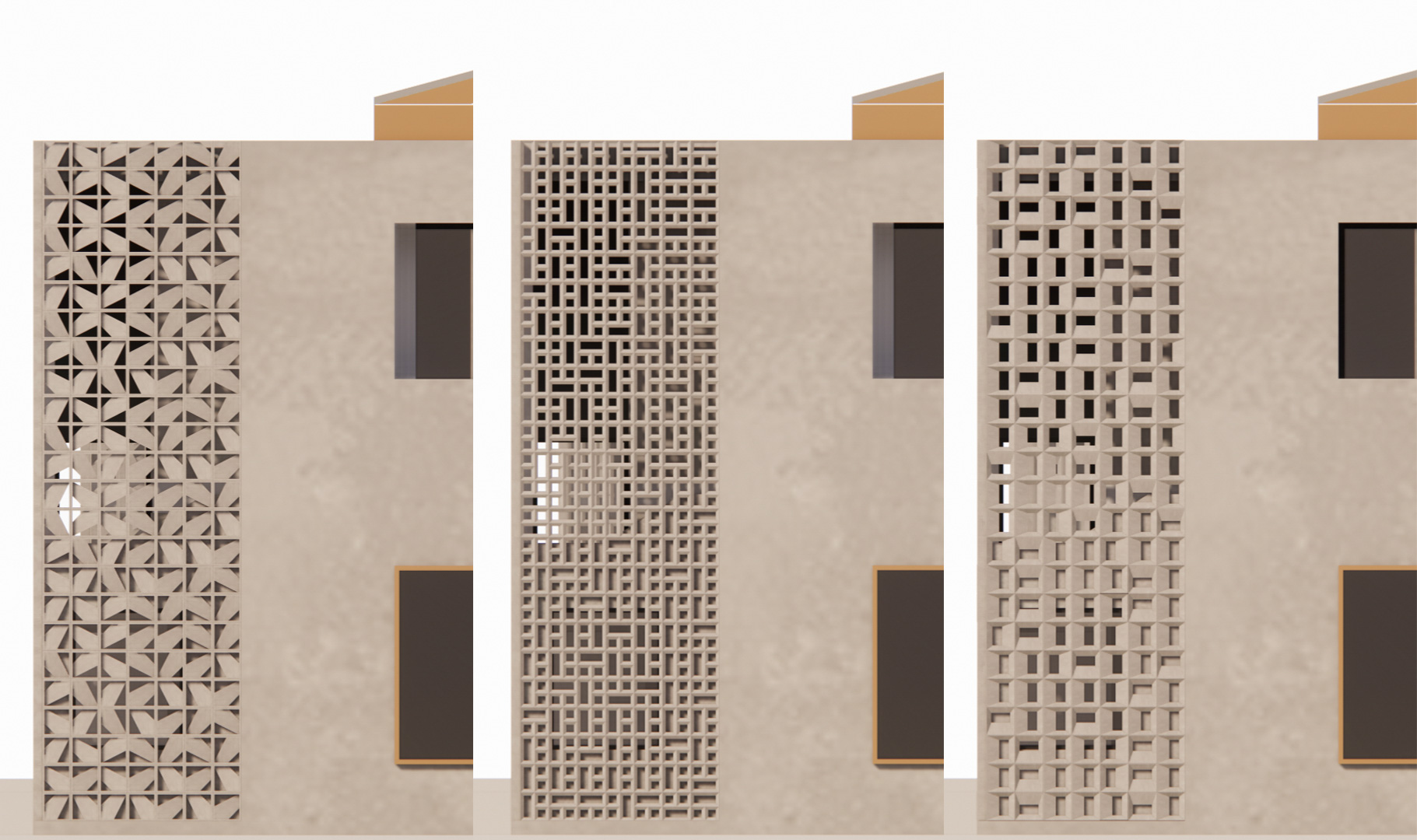
The incidence matrix of a non-binary design lists the number of times each element is repeated in each block. Often there are covariates in the experimental units that are known to affect the response variable and must be taken into account. Ideally an experimenter can group the experimental units into blocks where the within block variance is small, but the block to block variability is large. For example, in testing a drug to prevent heart disease, we know that gender, age, and exercise levels play a large role. We should partition our study participants into gender, age, and exercise groups and then randomly assign the treatment (placebo vs drug) within the group.
Messi Blocks FC Barcelona Star From Joining Inter Miami Reports El Nacional
In other words, good blocking variables decreases error, which increases statistical power. The block size refers to the number of experiment units in a block.Commonly block sizes are equal, denoted by \(b\).Sometimes the block sizesare naturally defined, and sometimes they need to be specificallyselected by the experimenter. In general, we are faced with a situation where the number of treatments is specified, and the block size, or number of experimental units per block (k) is given. This is usually a constraint given from the experimental situation.
Projective planes
However, this method of constructing a BIBD using all possible combinations, does not always work as we now demonstrate. If the number of combinations is too large then you need to find a subset - - not always easy to do. As an example, let's take any 3 columns from a 4 × 4 Latin Square design. There are two additional assumptions unique to randomized block ANOVA. A farmer possesses five plots of land where he wishes to cultivate corn.
Bargain Block's Keith Bynum & Evan Thomas On How To Design A Home On A Budget - Exclusive Interview - House Digest
Bargain Block's Keith Bynum & Evan Thomas On How To Design A Home On A Budget - Exclusive Interview.
Posted: Wed, 23 Aug 2023 07:00:00 GMT [source]
Symmetric 2-designs (SBIBDs)
The sets of similarexperimental units are grouped together to form blocks, and theconditions that vary from block to block form the levels of the blockingfactor. The intent of blocking is to prevent large differences in theexperimental units from masking differences between treatment effects,while at the same time allowing the treatments to be examined underdifferent experimental conditions. Randomized block design still uses ANOVA analysis, called randomized block ANOVA.
Block a few of the most important nuisance factors
Although models (1) and(2) are similar to the two-way main-effects modelwith two treatment factors and the two-way complete model, respectively,they differ from the two-way models involving two treatment factors. In a block design, theunits are grouped into blocks and treatments are randomly assignedwithin each block. This difference leads to some controversy as towhether or not a test of equality of block effects is valid. While it is true randomized block design could be more powerful than single-factor between-subjects randomized design, this comes with an important condition.
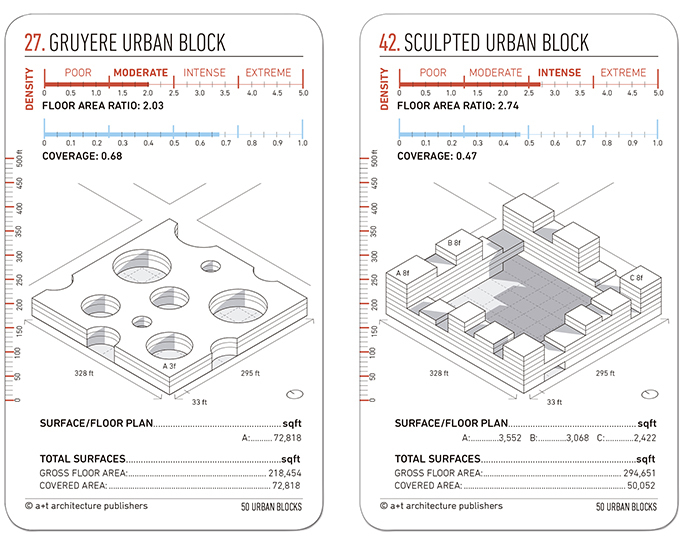
Identify nuisance variables
Both of these are integers, so if a design were to exist, each point would appear in \(7\) blocks, and there would be \(21\) blocks. We will not prove that such a design does not exist as the proof would be tedious and unenlightening. We will verify that the parameters satisfy the necessary conditions. Since there can’t be a fractional number of blocks, the second result follows. Similarly, given a BIBD\((v, k, λ)\), and a multigraph \(λK_v\), label the vertices of \(K_v\) with the points of the design. For each block of the design, use a new colour to colour the edges of a \(K_k\) that connects the points in that block.
In this example we will block on field since there might be differences in soil type, drainage, etc from field to field. In each field, we will plant all three varieties so that we can tell the difference between varieties without the block effect of field confounding our inference. Thus, if we know that a design is regular, uniform, and balanced, then the parameters \(r\) and \(b\) can be determined from the parameters \(v\), \(k\), and \(λ\). We therefore often shorten our notation and refer to a BIBD\((v, k, λ)\). Obtained from counting for a fixed x the triples (x, y, B) where x and y are distinct points and B is a block that contains them both. This equation for every x also proves that r is constant (independent of x) even without assuming it explicitly, thus proving that the condition that any x in X is contained in r blocks is redundant and r can be computed from the other parameters.
What is Blocking?
In the example above, the cell phone use treatment (yes vs. no) cannot interact with driving experience. This means the effect of cell phone use treatment (yes vs. no) on the dependent variable, driving ability, should not be influenced by the level of driving experience (seasoned, intermediate, inexperienced). In other words, the impact of cell phone use treatment (yes vs. no) on the dependent variable should be similar regardless of the level of driving experience. If this assumption is violated, randomized block ANOVA should not performed.
Use This Design Idea From HGTV's Bargain Block To Make Your Shower Feel Bigger - House Digest
Use This Design Idea From HGTV's Bargain Block To Make Your Shower Feel Bigger.
Posted: Sun, 17 Sep 2023 07:00:00 GMT [source]
One possible alternative is to treat it like a factorial ANOVA where the independent variables are allowed to interact with each other. In randomized block design, the control technique is done through the design itself. First the researchers need to identify a potential control variable that most likely has an effect on the dependent variable. Researchers will group participants who are similar on this control variable together into blocks. This control variable is called a blocking variable in the randomized block design. The purpose of the randomized block design is to form groups that are homogeneous on the blocking variable, and thus can be compared with each other based on the independent variable.
That would increase the experimental error of each resistivity measurement by the run-to-run furnace variability and make it more difficult to study the effects of the different dosages. The blocked way to run this experiment, assuming you can convince manufacturing to let you put four experimental wafers in a furnace run, would be to put four wafers with different dosages in each of three furnace runs. The only randomization would be choosing which of the three wafers with dosage 1 would go into furnace run 1, and similarly for the wafers with dosages 2, 3 and 4. Implementing blocking in experimental design involves a series of steps to effectively control for extraneous variables and enhance the precision of treatment effect estimates.
And then, the researcher must decide how many blocks are needed to run and how many replicates that provides in order to achieve the precision or the power that you want for the test. There are plenty of experimental designs where we have levels of treatments nested within each other for practical reasons. The literature often gives the example of an agriculture experiment where we investigate the effect of irrigation and fertilizer on the yield of a crop. However because our irrigation system can’t be fine-tuned, we have plots with different irrigation levels and within each plot we have perhaps four subplots that have the fertilizer treatment. To summarize, Irrigation treatments were randomly assigned to plots, and fertilizer treatments were randomly assigned to sub-plots.
This empowers creators to directly monetize their work while enabling transparent and automated reward distribution based on usage and performance. Another exciting application of generative AI in the blockchain domain is creating synthetic data for testing and validation. Blockchain systems, like the Ethereum network processing over 1 million transactions daily, often require extensive testing to ensure their robustness and reliability. However, obtaining real-world data for testing can be challenging due to privacy concerns and data scarcity. Generative AI can overcome this hurdle by generating realistic synthetic data that mimics real-world scenarios.
When we want to infer if the amount of noise explained by adding Irrigation or Fertilizer is sufficiently large to justify their inclusion into the model, we compare the sum-of-squares value to the RSS but now we have to use the appropriate pool. Next we can do the appropriate analysis for the fertilizer, recognizing that all the p-values for the plot effects are nonsense and should be ignored. Notice that in our block level, there is no p-value to assess if the blocks are different. So our analysis respects that blocks are present, but does not attempt any statistical analyses on them.
One of the primary concerns surrounding generative AI is the need for more transparency and accountability in creating and using these models. Blockchain’s inherent properties of immutability, traceability and transparency can help mitigate these issues. A study conducted in China found that generative AI can significantly enhance the security of blockchain networks by identifying potential vulnerabilities and suggesting improvements. For instance, the LLM4FUZZ technique, which combines large language models (LLMs) with fuzz testing methodologies, has shown promising results in detecting smart contract vulnerabilities.
No comments:
Post a Comment